
Whatsapp integrators
official partner
Start engaging with your customers where they already are.
Trusted by leading brands
WIP
GotBot & WhatsApp. Choose your plan.
Transforming
big business,
one conversation
at at time.
Focusing on the Financial services, Insurance,
Retail & Property sectors, GotBot Ai is here to take your business to new heights.
See our case studies below
Transforming
big business,
one conversation
at at time.
Focusing on the Financial services, Insurance,
Retail & Property sectors, GotBot Ai is here to take your business to new heights.
See our case studies below
Automate responses across multiple
messaging services from
a single dashboard.
Automate responses across multiple
messaging services from
a single dashboard.
Automate responses across multiple
messaging services from
a single dashboard.

What is Amazon Bedrock
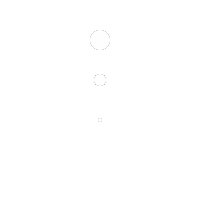
What sets Bedrock apart
Quick Overview
Amazon Bedrock is a fully managed service that offers a choice of high-performing foundation models (FMs). with a single API, along with a broad set of capabilities you need to build generative AI applications, simplifying development while maintaining privacy and security. You can easily experiment with a variety of top FMs, privately customize them with your data using techniques such as fine-tuning and retrieval augmented generation (RAG), and create managed agents that execute complex business tasks—from booking travel and processing insurance claims to creating ad campaigns and managing inventory—all without writing any code.
Generative AI refers to AI systems that can generate new content like images, text, video, etc. based on the patterns they have learned from large datasets.
Large language models are a type of generative AI model that is specifically trained on massive text datasets. This allows them to generate new coherent text by predicting the next word or sequence of words based on the context.
Key features
1. Advanced Natural Language Processing (NLP) Capabilities:
Bedrock's NLP capabilities are nothing short of extraordinary. The model's proficiency in understanding and generating human-like text opens up new possibilities for applications ranging from conversational agents to content creation.
2. State-of-the-Art Image Generation:
Equipped with state-of-the-art image generation algorithms, Bedrock excels in creating realistic and diverse visual content. From artistic endeavours to practical image-based applications, the potential applications are as vast as your imagination.
3. Deep Learning for Diverse Applications:
Bedrock isn't confined to a singular domain. Its versatility in applying deep learning techniques spans various industries and applications, making it a versatile solution for a wide array of challenges.
Foundation Models:

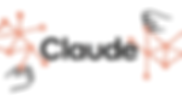
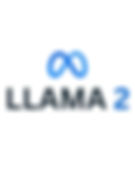
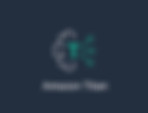
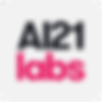

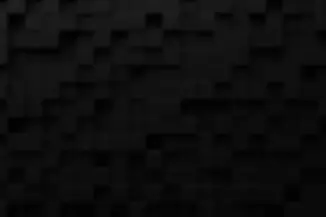
What are Large Language Models?
The term "large" refers to the scale and complexity of these models. They are typically trained on extensive datasets containing diverse text from the internet, books, articles, and other sources. This extensive training enables them to learn the patterns, grammar, and semantics of human language.
Large language models have a wide range of applications, including natural language processing (NLP) tasks such as text generation, completion, classification, translation, and question-answering. They can generate coherent and contextually appropriate text, understand complex language structures, and respond to queries in a human-like manner.
Some Large Language Models
Quick Overview




Human-Like Chatbots: Personalized Conversations
Improved Understanding
LLMs have a deeper understanding of context and semantics, enabling chatbots to comprehend user queries more accurately. This results in more relevant responses and reduces instances of misinterpretation
Efficiency and Scalability
Integrating an LLM automates the chatbot's response generation process, making it more efficient and scalable. Chatbots can handle a larger number of users simultaneously without compromising the quality of responses.
Multilingual Capabilities
LLMs are capable of understanding and generating text in multiple languages. Integrating an LLM with a chatbot allows it to communicate effectively with users from diverse linguistic backgrounds.
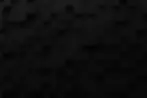
Bedrock model use cases:
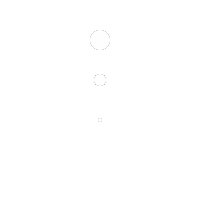

Anthropic:
Anthropic:
-
Sales and Customer Service: Claude acts as a virtual sales representative, enhancing customer interaction and satisfaction.
-
Business Analysis: Efficient in extracting and summarizing key information from business documents and emails.
-
Legal Document Processing: Assists in parsing legal documents for quick information retrieval, aiding legal professionals.
-
Coding and Technical Tasks: Continuously improving in coding, mathematical, and logical reasoning, making it a valuable asset for technical challenges.

Amazon Titan:
-
Text Models: Enhance productivity in tasks like blog post creation, article classification, open-ended Q&A, and conversational chat.
-
Multimodal Embeddings: Improve the accuracy of multimodal searches, recommendations, and personalization experiences.
-
Image Generator: Support content creators in generating high-quality images quickly and efficiently, ideal for industries like advertising, e-commerce, and media.

Stability.ai:
-
Personalized Advertising and Marketing Campaigns: Generating tailor-made ad visuals and marketing materials to enhance brand appeal.
-
Creative Asset Development: Ideating and crafting unlimited creative assets, including characters, scenes, and worlds, particularly useful in media, entertainment, gaming, and metaverse projects.

Meta Llama 2:
-
Llama-2-13b-chat: This version is adept at smaller-scale tasks like text classification, sentiment analysis, and language translation. Its 13B parameter size makes it suitable for applications that require precise, yet compact model capabilities.
-
Llama-2-70b-chat: Tailored for more extensive tasks like language modeling, text generation, and dialogue systems, the 70B parameter model excels in handling complex and large-scale AI challenges.

Al21 labs Jurassic:
-
Text generation for intricate tasks: Condense complex financial reports into succinct summaries and generates tailored financial and legal statements.
-
Natural language processing for real-time responses: Provide natural language responses to customer inquiries. Enable employees to access and interpret organisational data effortlessly.
-
Draft generation: Craft unique marketing content in various styles and lengths.

Cohere:
-
Perfect for knowledge assistants: Ideal for precise text retrieval, classification in knowledge management and information systems.
-
Text generation and content creation: Dynamic user experiences in customer support, content creation for marketing and media. Cost-effective for smaller-scale chat and content tasks, adaptable for business communications.
-
Classification, and clustering tasks: Multilingual applications in semantic search and data clustering for international business and research.